Artificial intelligence is always on everyone's lips. But how can AI actually be used efficiently? And how can sustainability be strengthened through AI? The Federal Network Agency's AI Café focused on efficiency through AI, rebound effects, possibilities and opportunities and specific use cases for AI. Our focus was on AI in energy management.
Use cases in energy management
Once a month, representatives from business, science and public authorities meet at the Federal Network Agency's AI Café, which in March focused on the topic of “Sustainability of and through AI”. As one of the four panelists, our Managing Director Dr. Thomas Goette spoke on the topic of “AI in energy management”.
Essentially, we focused on two points of contact for AI in energy management:
- Pattern recognition in the load profile
- Consumption Prediction
One is the automatic recognition of regular patterns in the consumption profile in order to identify optimizations and measures. The second is the prediction of consumption and consideration of various influencing factors for improved controlling and more savings.
Device disaggregation
As early as 2015 , we at GreenPocket worked with the Fraunhofer Institute on the NILM (Non-Intrusive Load Monitoring) research project to identify and allocate consumption to individual appliances and systems so that energy efficiency measures can be derived.
Automatic anomaly detection
Automatic anomaly detection is another result of the collaboration with the Fraunhofer IIS Institute. This determines a standard consumption based on historical consumption and automatically issues an alarm if consumption falls below or exceeds a certain tolerance range of this normal consumption. This allows excess consumption to be identified more quickly and avoids high costs. Creeping changes or damage to systems can also be detected more quickly and measures taken. This leads to savings in terms of budget and personnel.

The AI energy manager
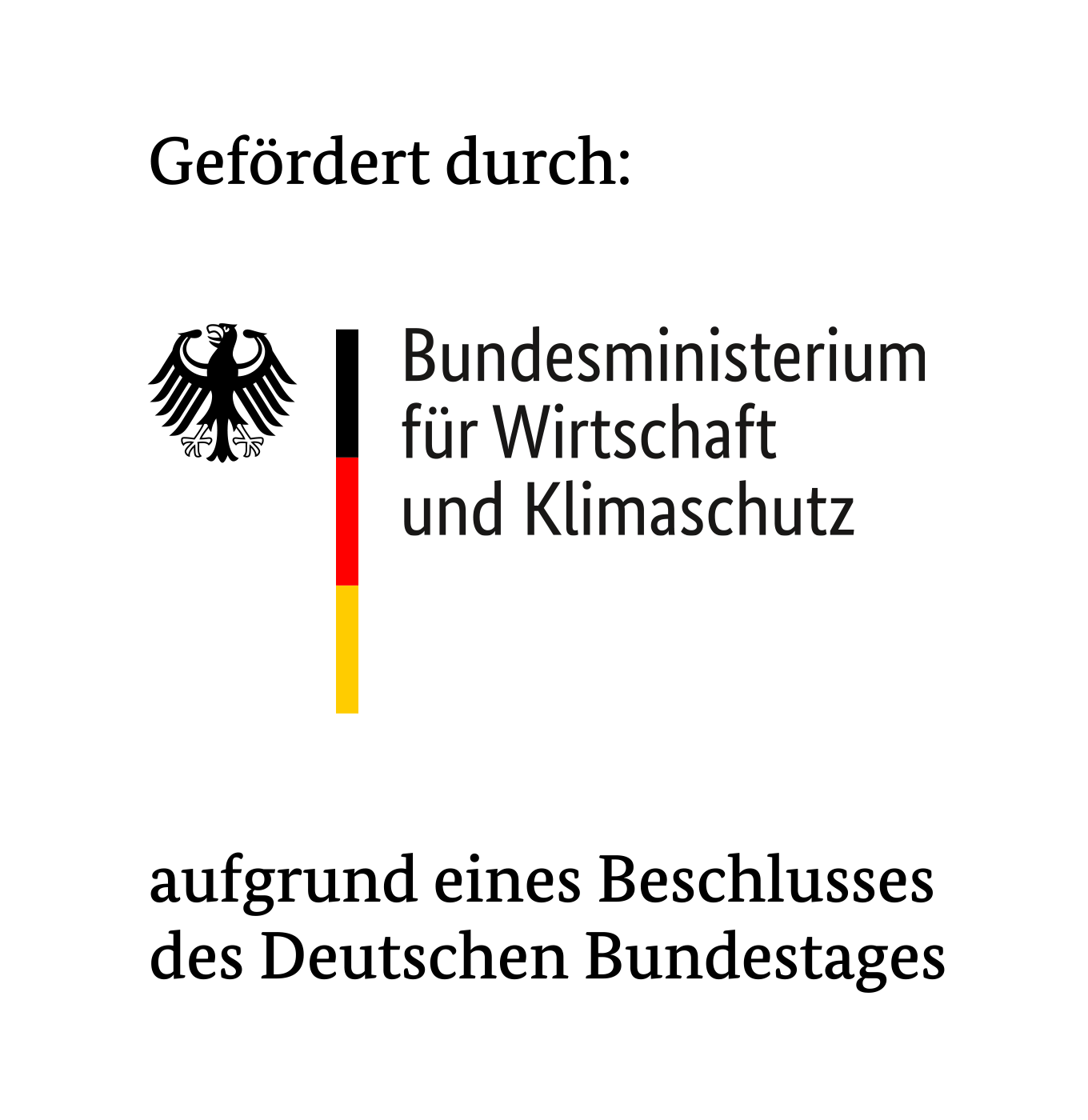
As part of the BMKW-funded energy-saving meter program, the energy management software is being further developed into AI energy management. This includes the automatic determination of the baseline, the AI-supported creation of key figures and the recommendation and review of measures.
Baseline determination requires consumption to be adjusted for various influencing factors, such as outside temperature, company size, opening hours or production volumes, because only by looking at an adjusted baseline can actual savings be made visible and not attributed to a smaller production volume, for example. This also makes it easier to track measures taken and check whether they have actually led to savings. The baseline determination can be used for any sector, e.g. electricity, gas or water.

Actual savings based on project examples
GreenPocket's AI energy manager is already being used by two project partners. One partner is a cash & carry store from the retail segment, the second is from the healthcare sector. Last year, the cash & carry store switched to LED lighting as an efficiency measure. The baseline comparison within the GreenPocket software showed that this measure led to savings of 8.2% last year compared to the previous year, taking into account opening hours, outside temperature and other temporal factors. At the hospital, the software was used to determine that the reparameterization of the heating system and the introduction of a demand-adjusted control system led to savings of 4.3% compared to the previous year. Influencing variables such as the outside temperature and the corona lockdown were also taken into account here.
Seven steps to becoming an AI energy manager
In order to be able to use the AI energy manager in a business, GreenPocket undertakes the following steps together with the project partner to achieve the highest quality results:
- Definition of a measurement concept to delimit the system to be examined in terms of energy
- Survey of external influencing factors that significantly influence energy use
- Import the time series data for the consumption data and influencing variables
- Define and implement energy efficiency measures
- Automatically or manually select the significant influencing variables on consumption
- Adjust the consumption and create a baseline (energy baseline)
- Use the baseline to determine the actual energy savings from a specific measure
Sustainability and AI - how do they go together?
The Federal Network Agency's AI Café was intended to highlight how AI can create more sustainability. Using AI in energy management is one of many things. Rebound effects were also discussed, as artificial intelligence requires high-performance data centers, which in turn account for a large proportion of total energy consumption. However, energy efficiency approaches are also continuously being developed here. As Dr. Michael Papen from Comma Soft rightly noted, “AI is a hammer, but not everything is a nail.” Even without artificial intelligence, there are enough algorithms that can automate processes and make them more efficient without the need for constant adaptation by artificial intelligence.
All in all, an exciting event about the possibilities of AI, security, hurdles, risks and prospects. Many thanks to all participants.